Dear Students and Colleagues:
We had a very successful ECE Capstone EXPO yesterday with 52 teams presenting their capstone projects. A panel of 50 judges from industry and academia joined us to select the top projects. In addition, three special awards (best in research, best in impact, and best in commercialization) were selected.
Speaking with the judges, I would like to report that they were very impressed with the quality of the capstone projects this year! The judges talked very highly about the complexity and innovation of the capstone projects and the enthusiasm with which they were presented. As a result, the judges decided to give awards to the top 15 teams this year instead of the usual top 10. I would like to thank our judges for their effort and time taken from their busy schedules to support our capstone program and celebrating our students' achievements!
Special thanks to the ECE Staff: Pam, Kevin, John, Arletta, Katie and Chris for helping to make this year’s EXPO such a success! This would not have been possible without their hard work and dedication, and months of planning in advance. My special thanks also to Prof. Demetrios Lambropoulos who has worked tirelessly from Fall 2023 to help and support the Capstone program. Many thanks also to all the students who volunteered in this event!
I would like to congratulate this year’s senior students who participated in the ECE capstone program and their advisors from inside and outside Rutgers ECE who helped guide their projects. Your help and support of our students are essential to the success of the Capstone Program!
I would like to acknowledge the support of the following industry sponsors: 7x24 Exchange Metro New York Chapter, Novo Nordisk, Lockheed Martin and L3Harris.
Here is the list of award recipients and their advisors:
Top 15 projects and Awardees of Best in Research, Commercialization and Social Impact:
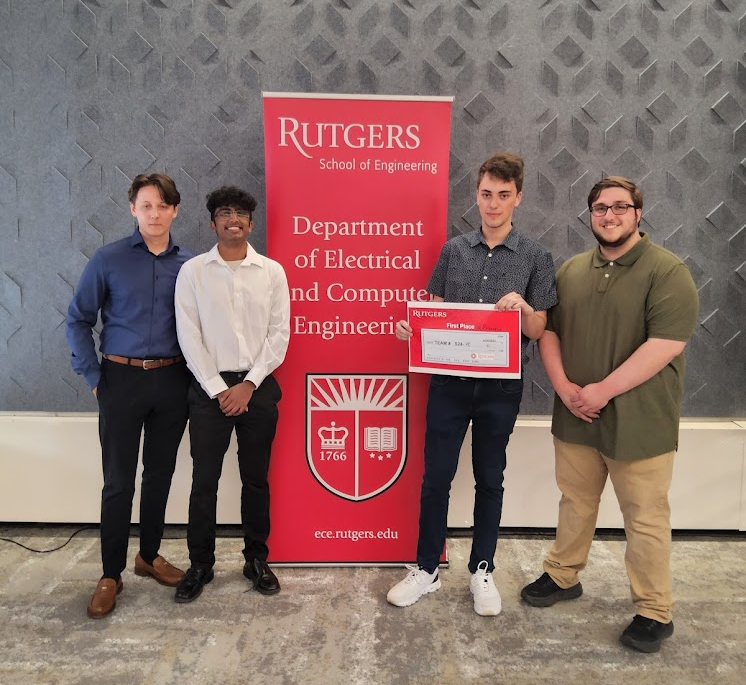
1st Place and Tie in Best in Research Award
Project S24-48: Radar Based Vital Sign Monitoring with Automated Beam Steering
Team members: Daniel Gore, Gavin Young, Felipe Valencia, Daniel Petronchak, Nithish Warren
Adviser: Dr. Athina Petropulu
Poster
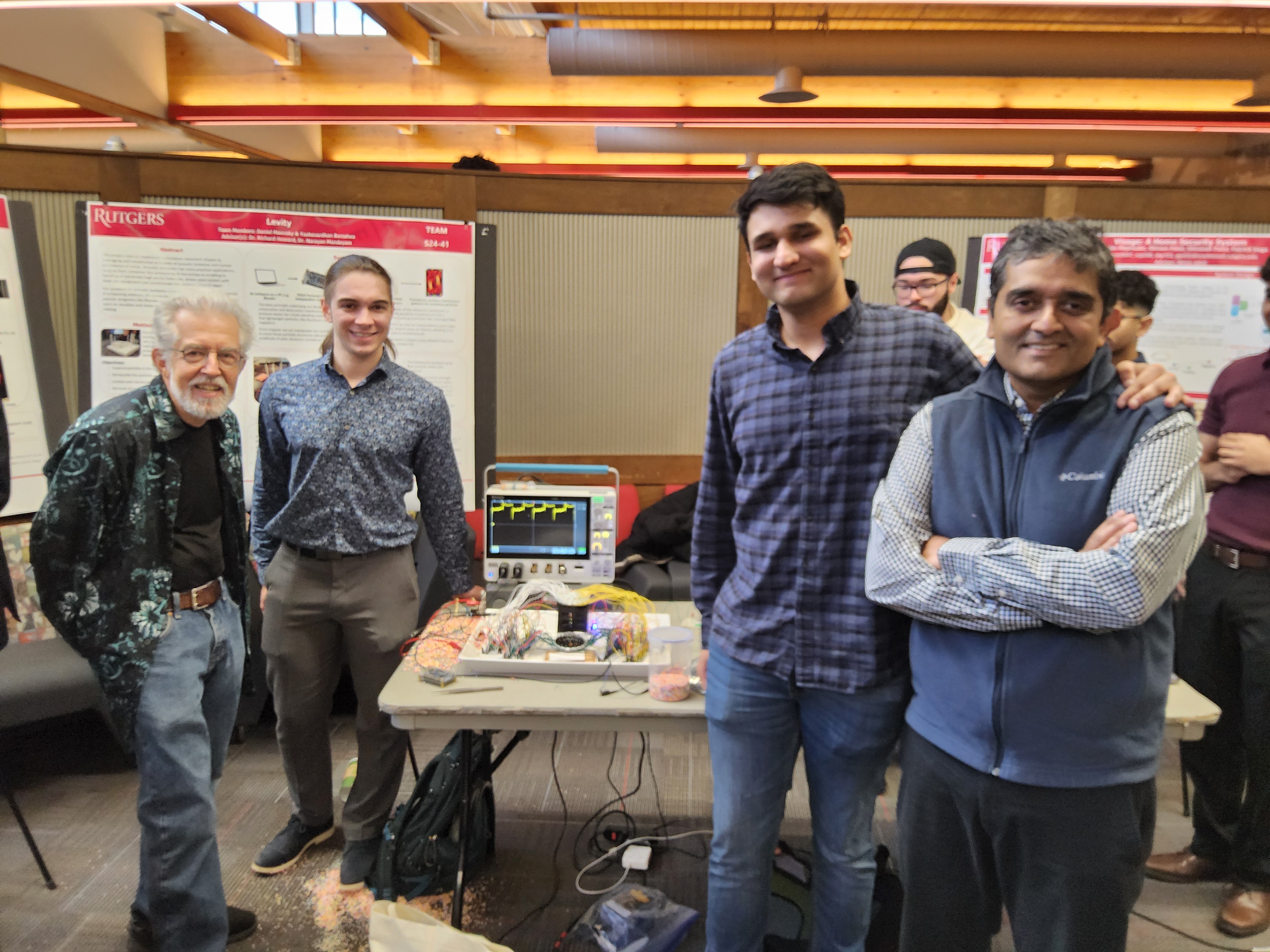
2nd Place and Tie in Best in Research Award
Project S24-41: Levity
Team members: Daniel Maevsky, Yashovardhan Bamalwa
Advisors: Dr. Richard Howard and Dr. Narayan Mandayam
Poster
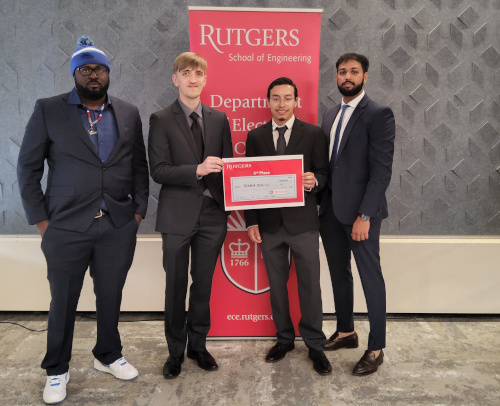
3rd Place
Project S24-02: RailVision: Overgrowth Detection Drone
Team members: Osmin Nolasco, James Sullivan, Steeve Cantave, Dhruv Patel
Advisors: Dr. Daniel Burbano Lombana & Dr. Sasan Haghani
Poster

4th Place
Project S24-24: Accessible Video Game Controller for One-Handed Individuals
Team members: Andrew Chacko, Teerth Patel, Mayank Barad, Georgiy Aleksanyan, Marco Ghabrial
Advisor: Dr. Jorge Ortiz
Poster

5th Place
Project S24-49: Cloud-Connected Automation and Optimization for Drones
Team members: Ravi Raghavan, Atharva Pandhare, Aryan Patil, Shreyas Ramachandran, Vijay Chandhar
Advisor: Dr. Maria Striki
Poster
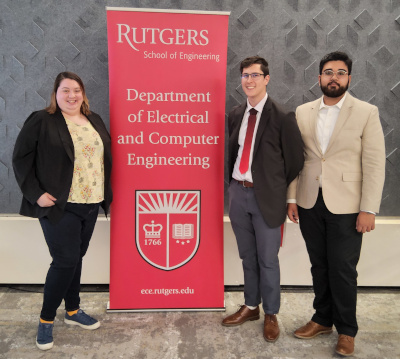
6th Place Tie and Best in Commercialization
Project S24-32: BlazeGuard : An Automated Fire Prevention Plug for Electronics and Appliances
Team members: Devesh Kaloty, Jason Peake, Taylor Scheuering
Advisor: Dr. Sasan Haghani and Don Bachman
Poster
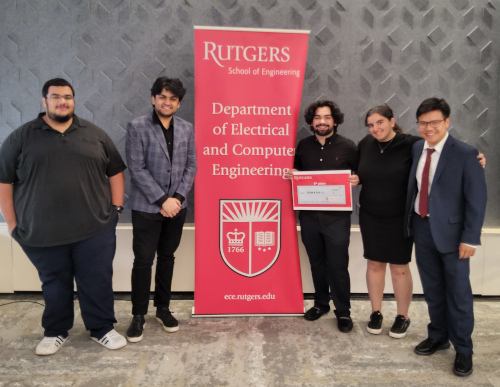
6th Place Tie and Tie in Best in Social Impact
Project S24-07: RINSIGHT - Real-time Interactive Neural Sensory Integration Glasses for Hearing Technology
Team members: William Ching, Jonathan Romero, Aliza Ezrapour, Aman Saxena, Matthew Gravatt
Advisor: Dr. Sheng Wei
Poster
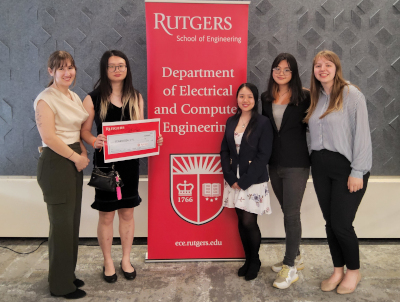
6th Place Tie
Project S24-54: HomeBud: Small-Scale Smart Watering System for Indoor Plants
Team members: Kasey Tian, Izabela Bigos, Victoria Chen, Kristina Jokic, Billie Liang
Advisor: Dr. Sasan Haghani
Poster

7th Place
Project S24-27: Cascaded-UNet: Medical Image Security Enhancement Through AI-Powered Digital Watermarking and Visual Cryptography
Team members: Fiona Wang, Zachary Asis, Yesmina Hammouda, Irina Mukhametzhanova, Thomas Trieu
Advisor: Dr. Dario Pompili and Tingcong Jiang
Poster

8th Place and Tie in Best Social Impact
Project S24-37: Human Motion Estimation for Interactive Rehabilitation
Team members: Ronan John, Daniel Gameiro, Marco Garcia-Palma
Advisor: Dr. Daniel Burbano Lombana
Poster

9th Place
Project S24-10: Design and Application of a Battery Management System for a Formula-Style Car
Team members: Mukund Ramakrishnan, Dana Fabiano, Ryan Billings, Thomas Troy Forzani, Daniel McCormack
Advisor: Dr. Michael Caggiano
Poster

10th Place
Project S24-31: SensoryNav
Team members: Ramya Ramabhadran, Raveena Gupta, Hima Nukala, Nandana Pai
Advisor: Dr. Jorge Ortiz
Poster

11th Place
Project S24-42: Hand ANalyzed Dynamics
Team members: Samuel Marran, James Artuso
Advisor: Dr. Sasan Haghani
Poster

12th Place
Project S24-03: Drone-Assisted Replication Training
Team members: Jinam Modasiya, Aaron Yagudaev, Keyur Rana, Ryan Meegan, Sean Maniar
Advisor: Dr. Laleh Najafizadeh and Dr. Laurent Burlion
Poster

13th Place
Project S24-29: Autonomous Parking Lot Navigation for Self-Driving Model Car
Team members: Oliver Rzepecki, Vraj Panchal, Isaiah Pajaro, Ryan Elizondo-Fallas
Advisor: Dr. Hang Liu
Poster

14th Place
Project S24-08: Ten(Sen)sor
Team members: Gurveer Grewal, Danial Fahim
Advisor: Demetrios Lambropoulos
Poster

15th Place
Project S24-46: Mini Busch Car
Team members: Aarushi Vashistha, Abraham Weitzman, Akash Gadicherla, Maanas Gopi, Peter Tran
Advisor: Dr. Sasan Haghani, Demetrios Lambropoulos & Jingang Yi
Poster
Congratulations to all the students and their advisors!
Best Regards,
Sasan Haghani
Undergraduate Program Director
Department of Electrical and Computer Engineering
Rutgers, The State University of New Jersey
94 Brett Rd, Piscataway, NJ, 08854
e-mail: sasan.haghani@rutgers.edu